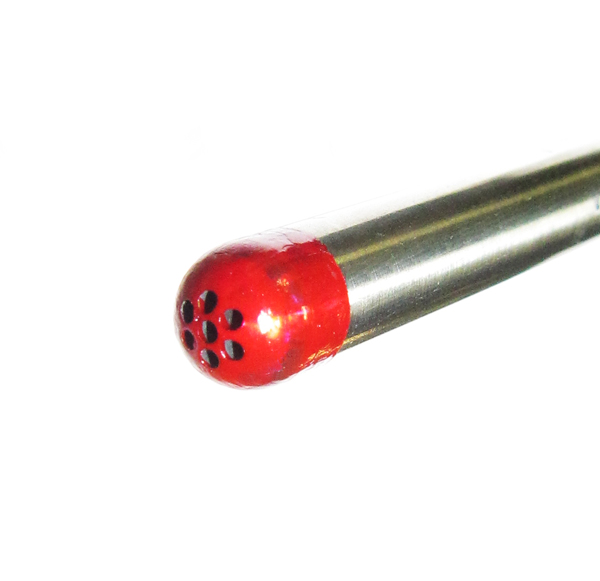
The weakest point in any multi-sensor probe system is almost always the data reduction process. Extracting the desired measured quantities from a large set of imperfect data points from different sensors will always be problematic- but, in the end, the process will usually boil down to a complex problem of interpolation. This is exactly the purpose for which machine learning approaches were developed.
In a recent research project, our Director of Research, Dr. David Birch, and his co-workers from the University of Surrey have applied some advanced neural network and machine learning techniques to the problem of converting signals from a seven-hole probe to a velocity magnitude and direction. Their work, described in a recent paper, has decreased the uncertainty and error introduced by the data reduction process by as much as 50% compared to the best available conventional multivariable interpolation techniques.
“This is clearly the next step in signal processing for these kinds of multi-sensor probe systems,” said Dr. Birch. “These machine-learning techniques can be used with any kind of data, and are very tolerant of measurement uncertainty. We calibrated our probe using not only the pressure sensors, but the environmental sensors as well- and the technique automatically corrected for sensitivity to ambient conditions”. The tests were carried out using the Surrey Sensors ID7HP-series probe, which includes ancillary sensors for absolute pressure, fluid temperature and humidity. All of these parameters were included in the data set used to “train” the machine-learning algorithm.
So, can we expect to see probes with this machine learning technology on the market any time soon? “The trick,” answered Dr. Paul Nathan, our Director of Technology, “is taking that proof-of-concept and making it small enough, fast enough and robust enough to be of use to our customers. That’s our next step.”